Compliance Monitor
Why consider machine learning for transaction monitoring?
The introduction of machine learning models alongside rules-based systems for transaction monitoring can significantly reduce alert volumes while also identifying cases of suspicious activity that would not have been found through the traditional rules-based method. This hybrid approach is gaining favour with regulators, say Priya Giuliani and Donald Andersen.
Priya Giuliani is a partner and Donald Andersen a senior director at Guidehouse's Financial Crime, Fraud and Investigative (FFI) Services (https://guidehouse.com). Contact them on pgiuliani@guidehouse.com and donald.andersen@guidehouse.com.
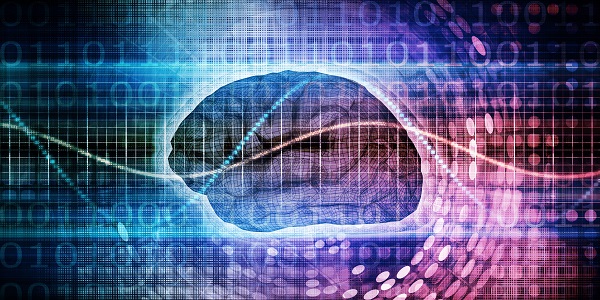
Advanced analytics has traditionally played an important role in Anti-Money Laundering (AML) compliance. Machine learning
(ML) represents the next evolution in advanced analytics and can be leveraged as part of AML compliance technology. While
many institutions are concerned about regulatory acceptance of ML in AML compliance, more familiarity with the technologies
and better understanding of the benefits is easing some of those initial concerns. In fact, a Guidehouse survey, 'The Evolving
Role of ML in Fighting Financial Crime', found that 77 per cent of European financial institutions (FIs) have begun to consider
using ML as part of their compliance technology. [1]